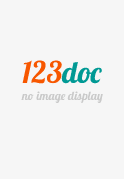
Đang tải... (xem toàn văn)
Thông tin tài liệu
Nghiên cứu giải pháp nâng cao chất lượng phân loại tín hiệu thủy âm tại vùng biển nước nông ứng dụng trí tuệ nhân tạo.Nghiên cứu giải pháp nâng cao chất lượng phân loại tín hiệu thủy âm tại vùng biển nước nông ứng dụng trí tuệ nhân tạo.Nghiên cứu giải pháp nâng cao chất lượng phân loại tín hiệu thủy âm tại vùng biển nước nông ứng dụng trí tuệ nhân tạo.Nghiên cứu giải pháp nâng cao chất lượng phân loại tín hiệu thủy âm tại vùng biển nước nông ứng dụng trí tuệ nhân tạo.Nghiên cứu giải pháp nâng cao chất lượng phân loại tín hiệu thủy âm tại vùng biển nước nông ứng dụng trí tuệ nhân tạo.Nghiên cứu giải pháp nâng cao chất lượng phân loại tín hiệu thủy âm tại vùng biển nước nông ứng dụng trí tuệ nhân tạo.Nghiên cứu giải pháp nâng cao chất lượng phân loại tín hiệu thủy âm tại vùng biển nước nông ứng dụng trí tuệ nhân tạo.Nghiên cứu giải pháp nâng cao chất lượng phân loại tín hiệu thủy âm tại vùng biển nước nông ứng dụng trí tuệ nhân tạo.Nghiên cứu giải pháp nâng cao chất lượng phân loại tín hiệu thủy âm tại vùng biển nước nông ứng dụng trí tuệ nhân tạo.Nghiên cứu giải pháp nâng cao chất lượng phân loại tín hiệu thủy âm tại vùng biển nước nông ứng dụng trí tuệ nhân tạo.Nghiên cứu giải pháp nâng cao chất lượng phân loại tín hiệu thủy âm tại vùng biển nước nông ứng dụng trí tuệ nhân tạo.Nghiên cứu giải pháp nâng cao chất lượng phân loại tín hiệu thủy âm tại vùng biển nước nông ứng dụng trí tuệ nhân tạo.Nghiên cứu giải pháp nâng cao chất lượng phân loại tín hiệu thủy âm tại vùng biển nước nông ứng dụng trí tuệ nhân tạo.Nghiên cứu giải pháp nâng cao chất lượng phân loại tín hiệu thủy âm tại vùng biển nước nông ứng dụng trí tuệ nhân tạo.Nghiên cứu giải pháp nâng cao chất lượng phân loại tín hiệu thủy âm tại vùng biển nước nông ứng dụng trí tuệ nhân tạo.Nghiên cứu giải pháp nâng cao chất lượng phân loại tín hiệu thủy âm tại vùng biển nước nông ứng dụng trí tuệ nhân tạo.Nghiên cứu giải pháp nâng cao chất lượng phân loại tín hiệu thủy âm tại vùng biển nước nông ứng dụng trí tuệ nhân tạo.Nghiên cứu giải pháp nâng cao chất lượng phân loại tín hiệu thủy âm tại vùng biển nước nông ứng dụng trí tuệ nhân tạo.Nghiên cứu giải pháp nâng cao chất lượng phân loại tín hiệu thủy âm tại vùng biển nước nông ứng dụng trí tuệ nhân tạo.Nghiên cứu giải pháp nâng cao chất lượng phân loại tín hiệu thủy âm tại vùng biển nước nông ứng dụng trí tuệ nhân tạo.Nghiên cứu giải pháp nâng cao chất lượng phân loại tín hiệu thủy âm tại vùng biển nước nông ứng dụng trí tuệ nhân tạo.Nghiên cứu giải pháp nâng cao chất lượng phân loại tín hiệu thủy âm tại vùng biển nước nông ứng dụng trí tuệ nhân tạo.Nghiên cứu giải pháp nâng cao chất lượng phân loại tín hiệu thủy âm tại vùng biển nước nông ứng dụng trí tuệ nhân tạo.Nghiên cứu giải pháp nâng cao chất lượng phân loại tín hiệu thủy âm tại vùng biển nước nông ứng dụng trí tuệ nhân tạo.Nghiên cứu giải pháp nâng cao chất lượng phân loại tín hiệu thủy âm tại vùng biển nước nông ứng dụng trí tuệ nhân tạo.Nghiên cứu giải pháp nâng cao chất lượng phân loại tín hiệu thủy âm tại vùng biển nước nông ứng dụng trí tuệ nhân tạo.Nghiên cứu giải pháp nâng cao chất lượng phân loại tín hiệu thủy âm tại vùng biển nước nông ứng dụng trí tuệ nhân tạo.
MINISTRYOFEDUCATIONANDTRAINING MINISTRY OFDEFENSE ACADEMY OF MILITARY SCIENCE AND TECHNOLOGY BACH NHAT HOANG RESEARCHING ON SOLUTION OF IMPROVING THE QUALITY OF UNDERWATER SIGNAL CLASSIFICATION IN SHALLOW WATERS APPLYING ARITIFICIAL INTELLIGENCE SUMMARY OF PhD THESIS IN ENGINEERING HANOI – 2023 MINISTRYOFEDUCATIONANDTRAINING MINISTRY OFDEFENSE ACADEMY OF MILITARY SCIENCE AND TECHNOLOGY BACH NHAT HOANG RESEARCHING ON SOLUTION OF IMPROVING THE QUALITY OF UNDERWATER SIGNAL CLASSIFICATION IN SHALLOW WATERS APPLYING ARITIFICIAL INTELLIGENCE Specialization: Electronic Engineering Code: 52 02 03 SUMMARY OF PhD THESIS IN ENGINEERING SCIENTIFIC SUPERVISORS: Assoc, Prof Dr Nguyen VanDuc Dr Vu LeHa HANOI – 2023 DECLARATION I hereby declare that this is my own research work under the guidance of my supervisors The statistics and results presented in this thesis are completely honest and have not been published in any other works References are fully cited Hanoi, April 12nd, 2023 Author Bach Nhat Hoang ACKNOWLEDGEMENT During the process of conducting research and completing this thesis, the PhD student has received valuable guidance, supports and comments, as well as sincere encouragements from scientists, teachers, colleagues and familym e m b e r s First of all, the PhD student wouldliketo express his gratitude to Assoc Prof Dr NguyenVanDuc and Dr.VuLe Ha for their enthusiastic guidance and support in the entire process of conducting research and completing the thesis Also, the PhD student would like to show his sincere thanks to the Board of Management of the Academy Military of Science and Technology, the Training Department and the Institute of Electronics for giving the PhD student all the favorable conditions to study, research and complete the thesis Additionally, the PhD student sincerely thank the teachers, scientists, and colleagues at the Academy Military of Science and Technology, the Institute of Electronics, and Hanoi University of Science and Technology, etc for instructing and providing valuable suggestions to the PhD student throughout the process of conducting this thesis Last but not least, the PhD student is thankful for the encouragement, support, sharing and sacrifice of his family that help the PhD student to overcome difficulties and achieve the research results in this thesis Author Bach Nhat Hoang TABLE OF CONTENTS LIST OF ABBREVIATIONS vii LISTOFTABLES xi LIST OF FIGURES xi INTRODUCTION CHAPTER 1.OVERVIEW ON THE CLASSIFICATION OF BI- OTIC AND ABIOTIC UNDERWATER SIGNALS IN SHALLOW WATERS 1.1 Biotic-Abioticunderwatersignalsanduderwatersignalclassification system inshallowwaters 1.1.1 Theocean an dacoust ic p r o paga tion ch ar ac te ri st i cs in sh al- 1.1.2 low waters BioticandAbioticsoundsources 11 1.1.3 Underwater signal detection system based on sonarprinciple 13 1.2 Classicalapproachesinunderwatersignalclassification 19 1.2.1 Time-frequencydomaintransformation 19 1.2.2 LOFARalgorithm 22 1.2.3 CMSalgorithm 23 1.2.4 DEMONalgorithm 24 1.3 Modernapproachesinunderwatersignalclassification 26 1.3.1 RestrictedBoltzmannMachine 27 1.3.2 Auto-Encoder 28 1.3.3 ConvolutionNeuralNetwork 29 1.4 Thecurrentstateofresearchinunderwatersignalclassificationandlimitation 1.4.1 Thesoleuseofartificialintelligence 30 32 1.4.2 Theu s e o f p r e processingcombinedwithartificialintelligence 1.4.3 Theuseoftransferlearning 35 1.4.4 39 Limitations 38 1.5 Research directions of the thesis and actual underwater datasets 40 1.5.1 Research directions ofthe thesis 41 1.5.2 Actualunderwaterdatasetsusedinthe thesis .43 1.6 Chapter1conclusion 48 CHAPTER 2.CLASSIFICATION OF PROPELLER SHIP SIGNALS USING THE SOLUTION OF PROPOSED SPEC- TRAL AMPLITUDEVARIATIONCOMBINED WITH A CUSTOMIZED CONVOLUTION NEURAL NETWORK 49 2.1 The formation process of the propeller ship signals duringmovement 49 2.1.1 Signals generated frommovingpropellerships 49 2.1.2 Cavitationphenomenon .51 2.2 Proposal of spectral amplitudevariationpre-processing .52 2.2.1 Drawbacks of theDEMONalgorithm 52 2.2.2 Mathematical analysis of thep r o p o s e d algorithm .54 2.2.3 Structure of thep r o p o s e d algorithm 57 2.2.4 Evaluationoftheproposedalgorithmonactualsh ipdata 60 2.2.5 Evaluation of the proposed algorithm on actual diverbreath data 66 2.3 Proposal of a customized convolutionneuralnetwork .69 2.3.1 Reasons for choosing convolutionneuralnetwork .69 2.3.2 Proposednetworkconfiguration 70 2.3.3 Evaluation of the proposed convolution neuralnetworkcom- plexity 74 2.4 Classification results using the combination oftwoproposedsolutions onpropellersignals 76 2.4.1 ClassificationresultsofDEMON-HilbertcombinedwithLeNet and VGG19 asc o n t r o l results 77 2.4.2 Classificationresultsoftheproposedspectralamplitudevari- ation algorithm with LeNeta n d VGG19 78 2.4.3 Classification results of DEMON-Hilbert and the proposed spectral amplitude variation algorithm combined with the proposedCNN .80 2.4.4 Evaluation of thep r o p o s e d network 83 2.5 Chapter2conclusion 85 CHAPTER 3.CLASSIFICATION OF MARINE MAMMAL AND PROPELLER SHIP SIGNALS USING THEPROPOSED SOLUTION OF CUBIC SPLINES INTERPOLATIONCOMBINED WITH PROBABILITY DIS- TRIBUTION IN THE HIDDENSPACEDOMAIN 88 3.1 Marine mammals communicationsignalstructure 88 3.2 Proposal of the cubic-splines interpolationpre-processing 89 3.2.1 Theoretical basis for usingc u b i c - s p l i n e s i n t e r p o l a t i o n 90 3.2.2 Interpolations on the frequency domain and proposed solutions9 3.2.3 Structure of thep r o p o s e d algorithm 95 3.2.4 Evaluation of the proposed algorithm on actual marinemammald at a 97 3.3 Proposal of probability distribution in the hidden space forSiamese triplelossnetwork .101 3.3.1 Structure of Siamese triplelossnetwork .101 3.3.2 Structure ofR e p - V G G m o d e l 102 3.3.3 Proposedso l u t i o n u sin g p r o b a b il it y d i str i b u t io n i nt h eh i d dens p a c e 103 3.3.4 Proposed solution usingSNN-VAEm o d e l 105 3.4 Classification results using the combination oftwoproposedsolutions on marine mammal andpropellersignals 108 3.4.1 Classification results of the proposedSNN-VAEon propeller signals 108 3.4.2 Classification results of the proposed cubic-splinesinterpolation andSNN-VAEon marinem a m m a l signals 113 3.4.3 Classification results of the proposed cubic-splinesinterpolation and SNN-VAE on marine mammal and propeller signals 119 3.5 Chapter3c o n cl u s io n 125 CONCLUSION 127 LIST OFSCIENTIFICPUBLICATION 130 REFERENCES 132 LIST OF ABBREVIATIONS Ak The last convolutionlayerfeaturemap c Speed of sound underwater( m / s ) C Number of inputc h a n n e l s E Orthogonalfunction E(z) Vectormean of random variablez f Signal frequency( H z ) F Windowsize of convolutionnetwork F(x) Spatial Signalsequence I Inputspectrogramsize L Totalglobalaverageweight L Loss function of Siamesenetwork nk(t) Cavitationnoise N0 /2 Powerspectraldensityofwhitenoise O^ Thesizeofthenetwork P Padding R Set of realn u m b e r s s(t) Noise generated from propellerb l a d e s S Stride Sa Salinity (perthousand) t Time of signal(second) Tk Propeller signalperiod Tp Temperature ofenviroment( C e l c i u s ) V(z) Vectorvariance of random variablez x(t) Signal at ther e c e i v e r zh The depth of the water( m e t e r ) αkc Weightof filter k when classifying classc γ Scale parameter on the timea x i s υ Position parameter on the timea x i s Ψλ,τ Waveletsfamiliesf u n c t i o n [.]∗ :=⟨⟩ Complexconjugation Equals function in the frequencydomain ≈ Approximately σk2 Powerspectraldensity λ Signalvariance [∗] Convolutionmultiplication τ Signaldelay ≜ Equals functionbydefinitionm a t h e m a t i c µB The mean of as u b s e t ϵ Random bias of convolutionalnetworks ACT Anisotropic chirplett r a n s f o r m s ADCNN Auditory perception inspired deep convolutional neuralnetworkAE Auto-encoder AG Arraygain AV Amplitudevariation BN BatchNorm BOC-NOAA Best of CUT-National Oceanic and Atmospheric Administration CMS Cyclic modulationS p e c t r u m CNN Convolution neuralnetworkCSDM Cross-spectral density matrices CSI Cubic-splinesinterpolation CWT Continuoswavelett r a n s f o r m DL Deeplearning DEMON Demodulation of envelope modulation on noise DT Detectionthreshold DWT Discretewavelett r a n s f o r m ELM Extreme learningm a c h i n e FC Fullyconnected FCNN Fullyconnected neuralnetworkFT FFT FourierTransform Fastfouriert r a n s f o r mNgày đăng: 30/05/2023, 06:51
Xem thêm:
Tài liệu cùng người dùng
Tài liệu liên quan