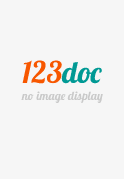
Đang tải... (xem toàn văn)
Thông tin tài liệu
Vibrations Fundamentals and Practice Appa Maintaining the outstanding features and practical approach that led the bestselling first edition to become a standard textbook in engineering classrooms worldwide, Clarence de Silva''s Vibration: Fundamentals and Practice, Second Edition remains a solid instructional tool for modeling, analyzing, simulating, measuring, monitoring, testing, controlling, and designing for vibration in engineering systems. It condenses the author''s distinguished and extensive experience into an easy-to-use, highly practical text that prepares students for real problems in a variety of engineering fields.
de Silva, Clarence W “Appendix A” Vibration: Fundamentals and Practice Clarence W de Silva Boca Raton: CRC Press LLC, 2000 Appendix A Dynamic Models and Analogies A system may consist of a set of interacted components or elements, each possessing an inputoutput (or cause-effect, or causal) relationship A dynamic system is one whose response variables are functions of time, with non-negligible rates of changes A more formal mathematical definition can be given, but it is adequate here to understand that vibrating systems are dynamic systems A model is some form of representation of a practical system An analytical model (or mathematical model) comprises a set of equations, or an equivalent, that approximately represents the system Sometimes, a set of curves, digital data (table) stored in a computer, and other numerical data — rather than a set of equations — can be termed an analytical model if such data represent the system of interest A model developed by applying a suitable excitation to a system and measuring the resulting response of the system is called an experimental model In general, then, models can be grouped into the following categories: Physical models (prototypes) Analytical models Computer (numerical) models Experimental models (using input/output experimental data) Mathematical definitions for a dynamic system are given with reference to an analytical model of the system; for example, a state-space model In that context, the system and its analytical model are synonymous In reality, however, an analytical model, or any model for that matter, is an idealization of the actual system Analytical properties that are established and results that are derived would be associated with the model rather than the actual system, whereas the excitations are applied to and the output responses are measured from the actual system This distinction should be clearly recognized Analytical models are very useful in predicting the dynamic behavior (response) of a system when it is subjected to a certain excitation (input) Vibration is a dynamic phenomenon and its analysis, practical utilization, and effective control require a good understanding of the vibrating system A recommended way to achieve this is through the use of a suitable model of the system A model can be employed for designing a mechanical system for proper vibration performance In the context of vibration testing, for example, analytical models are commonly used to develop test specifications, and also the input signal applied to the shaker, and to study dynamic effects and interactions in the test object, the shaker table, and their interfaces In product qualification by analysis, a suitable analytical model of the product replaces the test specimen In vibration control, a dynamic model of the vibrating system can be employed to develop the necessary control schemes (e.g., model-based control) Analytical models can be developed for mechanical, electrical, fluid, and thermal systems in a rather analogous manner because some clear analogies are present among these four types of systems In practice, a dynamic system can exist as a combination of two or more of the these various types, and is termed a mixed system In view of the analogy, then, a unified approach can be adopted in analysis, design, and control of these different types of systems and mixed systems In this context, understanding the analogies in different system types is quite useful in the development and utilization of models This appendix outlines some basic concepts of modeling Also, fundamental analogies that exist among different types of dynamic systems are identified ©2000 CRC Press A.1 MODEL DEVELOPMENT There are two broad categories of models for dynamic systems: lumped-parameter models and continuous-parameter models Lumped-parameter models are more commonly employed than continuous-parameter models, but continuous-parameter elements sometimes are included in otherwise lumped-parameter models in order to improve the model accuracy In lumped-parameter models, various characteristics in the system are lumped into representative elements located at a discrete set of points in a geometric space A coil spring, for example, has a mass, an elastic (spring) effect, and an energy-dissipation characteristic, each of which is distributed over the entire coil In an analytical model, however, these individual distributed characteristics can be approximated by a separate mass element, a spring element, and a damper element, which are interconnected in some parallel-series configuration, thereby producing a lumped-parameter model Development of a suitable analytical model for a large and complex system requires a systematic approach Tools are available to aid this process Signals (excitations and response) can be represented either in the frequency domain or in the time domain A time-domain model consists of a set of differential equations In the frequency domain, a model is represented by a set of transfer functions (or frequency-response functions) Frequency-domain transfer function considerations also lead to the concepts of mechanical impedance, mobility, and transmissibility The process of modeling can be made simple by following a systematic sequence of steps The main steps are summarized below: Identify the system of interest by defining its purpose and system boundaries Identify or specify the variables of interest These include inputs (forcing functions or excitations) and outputs (responses) Approximate (or model) various segments (or processes or phenomena) in the system by ideal elements, suitably interconnected Draw a free-body diagram for the system with suitably isolated components a Write constitutive equations (physical laws) for the elements b Write continuity (or conservation) equations for through variables (equilibrium of forces at joints; current balance at nodes, etc.) c Write compatibility equations for across (potential or path) variables These are loop equations for velocities (geometric connectivity), voltages (potential balance), etc d Eliminate auxiliary (unwanted) variables that are redundant and not needed to define the model Express system boundary conditions and response initial conditions using system variables These steps should be self-explanatory or integral with the particular modeling techniques A.2 ANALOGIES Analogies exist among mechanical, electrical, hydraulic, and thermal systems The basic system elements can be divided into two groups: energy-storage elements and energy-dissipation elements Table A.1 shows the linear relationships that describe the behavior of translatory mechanical, electrical, thermal, and fluid elements These relationships are known as constitutive relations In particular, Newton’s second law is considered the constitutive relation for the mass element The analogy used in Table A.1 between mechanical and electrical elements is known as the force–current analogy This analogy appears more logical than a force–voltage analogy, as is clear from Table A.2 This follows from the fact that both force and current are through variables, which are analogous to fluid flow through a pipe; and both velocity and voltage are across variables, which vary across ©2000 CRC Press TABLE A.1 Some Linear Constitutive Relations Constitutive Relation for Energy Dissipating Elements Energy Storage Elements A-Type (Across) Element System Type Translatory mechanical: v = Velocity f = Force Mass: Spring: dv = f dt (Newton’s second law) m = Mass Capacitor: df = kv dt (Hooke’s law) k = Stiffness Inductor: dv C =i dt C = Capacitance Thermal capacitor: di L =v dt L = Inductance None dT =Q dt Ct = Thermal capacitance Fluid capacitor: Fluid inertor: m Electrical: v = Voltage i = Current Thermal: T = Temperature difference Q = Heat transfer rate Fluid: P = Pressure difference Q = Volume flow rate T-Type (Through) Element Ct dP Cf =Q dt Cf = Fluid capacitance dQ If =P dt If = Inertance D-Type (Dissipative) Element Viscous damper: f = bv b = Damping constant Resistor: Ri = v R = Resistance Thermal Resistor: RtQ = T Rt = Thermal resistance Fluid resistor: RfQ = P Rf = Fluid resistance TABLE A.2 Force–Current Analogy System Type System-response variables: Through variables Across variables System parameters Mechanical Electrical Force f Velocity v m k b Current i Voltage v C 1/L 1/R the flow direction, as in the case of pressure The correspondence between the parameter pairs given in Table A.2 follows from the relations in Table A.1 Note that the rotational mechanical elements possess constitutive relations between torque and angular velocity, which can be treated as a generalized force and a generalized velocity, respectively In fluid systems as well, basic elements corresponding to capacitance (capacity), inductance (fluid inertia), and resistance (fluid friction) exist Constitutive relations between pressure difference and mass flow rate can be written for these elements In thermal systems, generally only two elements — capacitance and resistance — can be identified Constitutive relations exist between temperature difference and heat transfer rate in this case Proper selection of system variables is crucial in developing an analytical model for a dynamic system A general approach that can be adopted is to use across variables of the A-type (or acrosstype) energy storage elements and the through variables of the T-type (or through-type) energy ©2000 CRC Press storage elements as system variables (state variables) Note that if any two elements are not independent (e.g., if two spring elements are directly connected in series or parallel), then only a single state variable should be used to represent both elements Independent variables are not needed for D-type (dissipative) elements because their response can be represented in terms of the state variables of the energy storage elements (A-type and T-type) A.3 MECHANICAL ELEMENTS Here, one uses velocity (across variable) of each independent mass (A-type element) and force (through variable) of each independent spring (T-type element) as system variables (state variables) The corresponding constitutive equations form the “shell” of an analytical model These equations will directly lead to a state-space model of the system A.3.1 MASS (INERTIA) ELEMENT Constitutive equation (Newton’s second law): m dv = f dt (A.1) Since power = fv, the energy of the element is given by E= ∫ fvdt = ∫ m dt vdt = ∫ mvdv dv or Energy E = mv (A.2) This is the well-known kinetic energy Now, integrating equation (A.1), ( ) v( t ) = v − + m t ∫ fdt (A.3) − By setting t = 0+, one sees that ( ) ( ) v 0+ = v 0− (A.4) unless an infinite force f is applied to m Hence, one can state the following: Velocity can represent the state of an inertia element This is justified first because, from equation (A.3), the velocity at any time t can be completely determined with the knowledge of the initial velocity and the applied force, and because, from equation (A.2), the energy of an inertia element can be represented in terms of v alone Velocity across an inertia element cannot change instantaneously unless an infinite force/torque is applied to it A finite force cannot cause an infinite acceleration A finite instantaneous change (step) in velocity will need an infinite force Hence, v is a natural output (or state) variable and f is a natural input variable for an inertia element ©2000 CRC Press A.3.2 SPRING (STIFFNESS) ELEMENT Constitutive equation (Hook’s law): df = kv dt (A.5) Note that the conventional force-deflection Hooke’s law has been differentiated in order to be consistent with the variable (velocity) that is used with the inertia element As before, the energy is E= ∫ fvdt = ∫ f k dt dt = ∫ k fdf df or Energy E = f2 k (A.6) This is the well-known (elastic) potential energy Also, t ( ) + k ∫ vdt f (t ) = f − (A.7) 0− and hence, ( ) ( ) f 0+ = f 0− (A.8) unless an infinite velocity is applied to the spring element In summary, Force can represent the state of a stiffness (spring) element This is justified because the force of a spring at any general t can be completely determined with the knowledge of the initial force and the applied velocity, and also because the energy of a spring element can be represented in terms of f alone Force through a stiffness element cannot change instantaneously unless an infinite velocity is applied to it Force f is a natural output (state) variable and v is a natural input variable for a stiffness element A.4 ELECTRICAL ELEMENTS Here, one uses voltage (across variable) of each independent capacitor (A-type element) and current (through variable) of each independent inductor (T-type element) as system (state) variables A.4.1 CAPACITOR ELEMENT Constitutive equation: C ©2000 CRC Press dv =i dt (A.9) Since power is iv, the energy is E= ∫ ivdt = ∫ C dt vdt = ∫ Cvdv dv or Energy E = Cv (A.10) This is the electrostatic energy of a capacitor Also, ( ) v( t ) = v − + t ∫ idt C (A.11) − Hence, for a capacitor, ( ) ( ) v 0+ = v 0− (A.12) unless an infinite current is applied to a capacitor In summary, Voltage is an appropriate response variable (or state variable) for a capacitor element Voltage across a capacitor cannot change instantaneously unless an infinite current is applied Voltage is a natural output variable and current is a natural input variable for a capacitor A.4.2 INDUCTOR ELEMENT Constitutive equation: L di =v dt Energy E = (A.13) Li (A.14) This is the electromagnetic energy of an inductor Also, ( ) i (t ) = i − + L t ∫ vdt (A.15) − Hence, for an inductor, ( ) ( ) i 0+ = i 0− unless an infinite voltage is applied In summary, ©2000 CRC Press (A.16) Current is an appropriate response variable (or state variable) for an inductor Current through an inductor cannot change instantaneously unless an infinite voltage is applied Current is a natural output variable and voltage is a natural input variable for an inductor A.5 THERMAL ELEMENTS Here, the across variable is temperature (T) and the through variable is the heat transfer rate (Q) The thermal capacitor is the A-type element There is no T-type element in a thermal system The reason is clear There is only one type of energy (thermal energy) in a thermal system, whereas there are two types of energy in mechanical and electrical systems A.5.1 THERMAL CAPACITOR Consider a material control volume V, of density ρ, and specific heat c Then, for a net heat transfer rate Q into the control volume, one obtains dT dt (A.17) dT =Q dt (A.18) Q = ρvc or Ct where Ct = ρvc is the thermal capacitance of the control volume A.5.2 THERMAL RESISTANCE There are three basic processes of heat transfer: Conduction Convection Radiation There is a thermal resistance associated with each process, given by their constitutive relations as given below Conduction: Q= kA T ∆x (A.19) where k = conductivity A = area of cross section of the heat conduction element ∆x = length of heat conduction with a temperature drop of T The conductive resistance is Rk = ©2000 CRC Press ∆x kA (A.20) Q = hc AT Convection: (A.21) where hc = convection heat transfer coefficient A = area of heat convection surface with temperature drop of T The convective resistance is Rc = hc A ( Q = σFE FA A T14 − T24 Radiation: (A.22) ) (A.23) where σ FE FA A = = = = Stefan-Boltzmann constant effective emmisivity of the radiation source (of temperature T1) shape factor of the radiation receiver (of temperature T2) effective surface area of the receiver This corresponds to a nonlinear thermal resistor A.6 FLUID ELEMENTS Here, one uses pressure (across variable) of each independent fluid capacitor (A-type element) and volume flow rate (through variable) of each independent fluid inertor (T-type element) as system (state) variables A.6.1 FLUID CAPACITOR The heat transfer rate is Cf dP =Q dt (A.24) Note that a fluid capacitor stores potential energy (a “fluid spring”) unlike the mechanical A-type element (inertia), which stores kinetic energy For a liquid control volume V of bulk modulus β, the fluid capacitance is given by Cbulk = V β (A.25) For an isothermal (constant temperature, slow-process) gas of volume V and pressure P, the fluid capacitance is Ccomp = ©2000 CRC Press V P (A.26) For an adiabatic (zero heat transfer, fast process) gas, the capacitance is V kP Ccomp = (A.27) where k= cp (A.28) cv which is the ratio of specific heats at constant pressure and constant volume For an incompressible fluid in a container of flexible area A and stiffness k, the capacitance is Celastic = A2 k (A.29) Note: For a fluid with bulk modulus, the equivalent capacitance would be Cbulk + Celastic For an incompressible fluid column with an area of cross section A and density ρ, the capacitance is A ρg (A.30) dQ =P dt (A.31) Cgrav = A.6.2 FLUID INERTOR If This represents a T-type element However, it stores kinetic energy, unlike the mechanical T-type element (spring), which stores potential energy For a flow with uniform velocity distribution across an area A and over a length segment ∆x, the fluid inertance is given by If = ρ ∆x A (A.32) For a non-uniform velocity distribution, I f = αρ ∆x A (A.33) where a correction factor α has been introduced For a flow of circular cross section with a parabolic velocity distribution, use α = 2.0 ©2000 CRC Press A.6.3 FLUID RESISTANCE For the approximate, linear case: P = Rf Q (A.34) The more general, nonlinear case is given by P = K RQ n (A.35) where KR and n are parameters of the nonlinearity For viscous flow through a uniform pipe, one obtains R f = 128µ ∆x πd (A.36) ∆x wb (A.37) for a circular cross-section of diameter d, and R f = 12µ for a rectangular cross section of height b that is much smaller than its width w Also, µ is the absolute viscosity (or dynamic viscosity) of the fluid, and is related to the kinematic viscosity υ through µ = υρ (A.38) A.6.4 NATURAL OSCILLATIONS Mechanical systems can produce natural oscillatory responses (or free vibrations) because they can possess two types of energy (kinetic and potential) When one type of stored energy is converted to the other type repeatedly, back and forth, the resulting response is oscillatory Of course, some of the energy will dissipate (through the dissipative mechanism of damper) and the free natural oscillations will decay as a result Similarly, electrical circuits and fluid systems can exhibit natural oscillatory responses due to the presence of two types of energy storage mechanism, where energy can “flow” back and forth repeatedly between the two types of elements However, thermal systems have only one type of energy storage element (A-type) with only one type of energy (thermal energy) Hence, purely thermal systems cannot naturally produce oscillatory responses unless forced by external means, or integrated with other types of systems (e.g., fluid systems) A.7 STATE-SPACE MODELS More than one variable might be needed to represent the response of a dynamic system There also could be more than one input variable A time-domain analytical model is a set of differential equations relating the response variables to the input variables This set of system equations is generally coupled, so that more than one response variable appears in each differential equation A particularly useful time-domain representation for a dynamic system is a state-space model In this representation (state-space representation), an nth-order system is represented by n first-order differential equations, which generally are coupled This is of the general form: ©2000 CRC Press dq1 = f1 (q1 , q2 , K, qn , r1 , r2 , K, rm , t ) dt dq2 = f2 (q1 , q2 , K, qn , r1 , r2 , K, rm , t ) dt (A.39) M dqn = fn (q1 , q2 , K, qn , r1 , r2 , K, rm , t ) dt The n state variables can be expressed as the state vector [ q = q1 , q2 , K, qn ] T (A.40) which is a column vector Note that [ ]T denotes the transpose of a matrix or vector The space formed by all possible state vectors of a system is the state space At this stage, one may wish to review the concepts of linear algebra given in Appendix C The state vector of a dynamic system is a least set of variables that is required to completely determine the state of the system at all instants of time They may or may not have a physical interpretation The state vector is not unique; many choices are possible for a given system Output (response) variables of a system can be completely determined from any such choice of state variables Since the state vector is a least set, a given state variable cannot be expressed as a linear combination of the remaining state variables in that state vector One suitable choice of state variables is the across variables of the independent A-type energy-storage elements and through variables of the independent T-type energy-storage elements The m variables r1, r2, …, rm in equations (A.39) are input variables, and they can be expressed as the input vector: [ r = r1 , r2 , K, rm ] T (A.41) Now, equation (A.39) can be written in the vector notation q˙ = f (q, r , t ) (A.42) When t is not present explicitly in the function f, the system is said to be autonomous A.7.1 LINEARIZATION Equilibrium states of the dynamic system given by equation (A.42), correspond to q˙ = (A.43) Consequently, the equilibrium states q are obtained by solving the set of n algebraic equations f (q, r , t ) = (A.44) for a special steady input r Usually, a system operates in the neighborhood of one of its equilibrium states This state is known as its operating point The steady state of a dynamic system is also an equilibrium state ©2000 CRC Press Suppose that a slight excitation is given to a dynamic system that is operating at an equilibrium state If the system response builds up and deviates further from the equilibrium state, the equilibrium state is said to be unstable If the system returns to the original operating point, the equilibrium state is stable If it remains at the new state without either returning to the equilibrium state or building up the response, the equilibrium state is said to be neutral To study the stability of various equilibrium states of a nonlinear dynamic system, it is first necessary to linearize the system model about these equilibrium states Linear models are also useful in analyzing nonlinear systems when it is known that the variations of the system response about the system operating point are small in comparison to the maximum allowable variation (dynamic range) Equation (A.42) can be linearized for small variations δq and δr about an equilibrium point (q, r) by employing up to only the first derivative term in the Taylor series expansion of the nonlinear function f The higher-order terms are negligible for small δq and δr This method yields δq˙ = ∂f ∂f (q , r , t )δq + (q , r , t )δr ∂q ∂r (A.45) The state vector and input vector for the linearized system are denoted by [ ] [ ] δq = x = x1 , x , K, x n δr = u = u1 , u2 , K, um T (A.46) T (A.47) The linear system matrix A(t) and the input gain matrix B(t) are defined as A(t ) = ∂f (q , r , t ) ∂q (A.48) B(t ) = ∂f (q , r , t ) ∂r (A.49) Then, the linear state model can be expressed as x˙ = Ax + Bu (A.50) If the dynamic system is a constant-parameter system, or if it can be assumed as such for the time period of interest, then A and B become constant matrices A.7.2 TIME RESPONSE Time variation of the state vector of a linear, constant-parameter dynamic system can be obtained using the Laplace transform method The Laplace transform of equation (A.50) is given by sX (s) − x(0) = AX (s) + BU (s) (A.51) Consequently, x(t ) = ᏸ −1 (sI − A) x(0) + ᏸ −1 (sI − A) BU (s) −1 ©2000 CRC Press −1 (A.52) in which I denotes the identity (unit) matrix Note that ᏸ–1 denotes the inverse Laplace transform operator The square matrix (sI – A)–1 is known as the resolvent matrix Its inverse Laplace transform is the state-transition matrix: Φ(t ) = ᏸ −1 (sI − A) −1 (A.53) It can be shown that Φ(t) is equal to the matrix exponential Φ(t ) = exp( At ) = I + At + 2 A t +K 2! (A.54) The state-transition matrix can be analytically determined as a closed-form matrix function by the direct use of inverse transformation on each term of the resolvent matrix, using equation (A.53), or as a series solution using equation (A.54) One can reduce the infinite series given in equation (A.54) into a finite matrix polynomial of order n – using the Cayley-Hamilton theorem This theorem states that a matrix satisfies its own characteristic equation The characteristic polynomial of A can be expressed as ∆(λ ) = det( A − λI ) = an λn + an−1λn−1 + L + a0 (A.55) in which det( ) denotes determinant The notation ∆( A) = an An + an−1 An−1 + L + a0 I (A.56) is used Then, by the Cayley-Hamilton equation, one obtains = an An + an−1 An−1 + L + a0 I (A.57) To get a polynomial expansion for exp(At), one can write exp( At ) = S( A) ⋅ ∆( A) + α n−1 An−1 + α n−2 An−2 + L + α I (A.58) in which S(A) is an appropriate infinite series Since ∆(A) = by the Cayley-Hamilton theorem, however, one has exp( At ) = α n−1 An−1 + α n−2 An−2 + L + α I (A.59) Now, it is just a matter of determining the coefficients α0, α1, …, αn–1, which are functions of time This is done as follows From equation (A.58), exp(λt ) = S(λ ) ⋅ ∆(λ ) + α n−1λn−1 + α n−2 λn−2 + L + α (A.60) If λ1, λ2, …, λn are the eigenvalues of A, however, then, by definition, ∆(λ i ) = det( A − λ i I ) = ©2000 CRC Press for i = 1, 2, K, n (A.61) Thus, from equation (A.60), one obtains exp(λ i t ) = α n−1λni −1 + α n−2 λni −2 + L + α for i = 1, 2, K, n (A.62) If the eigenvalues are all distinct, equation (A.62) represents a set of n independent algebraic equations from which the n unknowns α0, α1, …, αn–1 can be determined Because the product in the Laplace domain is a convolution integral in the time domain, and vice versa, the second term on the right-hand side of equation (A.52) can be expressed as a matrix convolution integral This gives t ∫ x(t ) = Φ(t )x(0) + Φ(t − τ) Bu( τ)dτ (A.63) The first part of this solution is the zero-input response; the second part is the zero-state response State variables are not necessarily measurable and generally are not system outputs A linearized relationship between state variables and system output (response) variables y(t) can be expressed as y(t ) = Cx(t ) (A.64) in which the output vector is [ y = y1 , y2 , K, y p ] T (A.65) and C denotes the output (measurement) gain matrix When m > and p > 1, the system is said to be a multi-input–multi-output (MIMO) system Note that, in this case, one has a transfer matrix H(s) given by H (s) = C(sI − A) B (A.66) Y (s) = H (s)U (s) (A.67) −1 which satisfies Since (sI − A)−1 = adj(sI − A) det(sI − A) (A.68) in which adj( ) denotes the adjoint (see Appendix C) It is seen that the poles, or eigenvalues, of the system (matrix A) are given by the solution of its characteristic equation: det(sI − A) = which should be compared with equation (A.61) If all eigenvalues of A have negative real parts, then the state-transition matrix Φ(t) in equation (A.63) will be bounded as t → ∞, which means that the linear system is stable ©2000 CRC Press A.7.3 SOME FORMAL DEFINITIONS A state vector x is a column vector that contains a minimum set of state variables x1, x2, …, xn that completely determines the state of a dynamic system The number of state variables, n, is the order of the system This is typically equal to the number of independent energy storage elements in the system, and is twice the number of degrees of freedom (for a mechanical system) Property 1: If the state vector x(t0) at the time t0 and the input (forcing excitation) u[t0, t1] over the time interval [t0, t1] are known, where t1 is any future time, then x(t1) can be uniquely determined In other words, a transformation g can be defined such that ( [ x(t1 ) = g t0 , t1 , x(t0 ), u t0 , t1 ]) (A.69) By this property it should be clear that the order of a dynamic system is equal to the number of independent initial conditions needed to completely determine the system response Note that according to the causality of dynamic systems, future states cannot be determined unless the inputs up to that future time are known This means that the transformation g is nonanticipative Each forcing function u[t0, t1] defines a state trajectory The n-dimensional vector space formed by all possible state trajectories is known as the state space Property 2: If the state x(t1) and the input u(t1) are known at any time t1, the system response (output) vector y(t1) can be uniquely determined This can be expressed as ( ) y(t1 ) = h t1 , x(t1 ), u(t1 ) (A.70) Note that the transformation h has no memory in the sense that the response at a previous time cannot be determined through the knowledge of the present state and input Note also that, in general, system outputs are not identical to the states although the former can be uniquely determined by the latter A.7.4 ILLUSTRATIVE EXAMPLE A torsional dynamic model of a pipeline segment is shown in Figure A.1(a) Free-body diagrams in Figure A.1(b) show internal torques acting at sectioned inertia junctions for free motion A state model is obtained using the generalized velocities (angular velocities Ωi) of the independent inertia elements and the generalized forces (torques Ti) of the independent elastic (torsional spring) elements as state variables The minimum set of states that is required for a complete representation determines the system order There are two inertia elements and three spring elements — a total of five energy-storage elements The three springs are not independent, however The motion of two springs completely determines the motion of the third This indicates that the system is a fourth-order system One obtains the model as follows Newton’s second law gives ˙ = −T + T I1Ω 1 ˙ = −T − T I2 Ω 2 Hooke’s law gives T˙1 = k1Ω1 T˙2 = k2 (Ω − Ω1 ) ©2000 CRC Press FIGURE A.1 (a) Dynamic model of a pipeline segment, and (b) free-body diagrams Torque T3 is determined in terms of T1 and T2, using the displacement relation (compatibility) for the inertia I2: T1 T2 T3 + = k1 k2 k3 The state vector is chosen as x = [Ω1 , Ω , T1 , T2 ] T The corresponding system matrix is A= k1 − k 0 k1 I1 k − 3 I2 k1 0 − k 1 − 1 + I2 k2 I1 The output-displacement vector is T T T y= 1, + 2 k1 k1 k2 ©2000 CRC Press T which corresponds to the following output-gain matrix: 0 C= 0 0 k2 k1 k1 For the special case given by I1 = I2 = I and k1 = k3 = k, the system eigenvalues are λ , λ1 = ± jω1 = ± j k I λ , λ = ± jω = ± j k + k2 I and the corresponding eigenvectors are X1 , X1 = R1 ± jI1 = α1 T ω1 , ω1 , m jk1 , 0] [ X2 , X2 = R2 ± jI2 = α2 T ω , − ω , m jk1 , ± jk2 ] [ The modal contributions to the displacement vector are 1 Y1 = α1 sin ω1t 1 and 1 Y2 = α sin ω t −1 The mode shapes are given by the vectors S1 = [1, 1]T and S2 = [1, –1]T, which are sketched in Figure A.2 In general, each modal contribution introduces two unknown parameters, αi and φi, into the free response (homogeneous solution), where φi are the phase angles associated with the sinusoidal terms For an n-degree-of-freedom (order-2n) system, this results in 2n unknowns, which require the 2n initial conditions x(0) Further developments of modal analysis for lumped-parameter systems are found in Chapter A.7.5 CAUSALITY AND PHYSICAL REALIZABILITY Consider a dynamic system represented by the single input-output differential equation: dny d n−1 y d mu d m−1u a a y b b + + K + = + + K + b0 u m −1 m n −1 dt m dt m−1 dt n dt n−1 ©2000 CRC Press (A.71) FIGURE A.2 Mode shapes of the pipeline segment where y and u are the output and the input, respectively The causality (cause-effect) of this system should dictate that u is the input and y is the output Its transfer function is given by G( s ) = N (s) bm s m + bm−1s m−1 + K + b0 = s n + an−1s n−1 + K + a0 ∆( s) (A.72) Note that n is the order, ∆(s) is the characteristic polynomial, and N(s) is the numerator polynomial of the system Suppose that m > n Then, if one integrates equation (A.71) n times, one obtains y and its integrals on the LHS, but the RHS will contain at least one derivative of u Since the derivative of a step function is an impulse, this implies that a finite change in input will result in an infinite change in the response This is not physically realizable It follows that a physically realizable system cannot have a numerator order greater than the denominator order in its transfer function If, in fact, m > n, then what this means physically is that y should be the system input and u should be the system output In other words, the causality should be reversed in this case Furthermore, for a physically realizable system, a simulation block diagram can be established using integrals alone, without the need for derivatives (s) Note that pure derivatives are physically not s realizable If m > n, the simulation block diagram will need at least one derivative for linking u to y That will not be physically realizable, again, because it would imply the possibility of producing an infinite response by a finite input In other words, feed-forward paths with pure derivatives will not be needed in a simulation block diagram of a physically realizable system ©2000 CRC Press ... develop test specifications, and also the input signal applied to the shaker, and to study dynamic effects and interactions in the test object, the shaker table, and their interfaces In product... excitation (input) Vibration is a dynamic phenomenon and its analysis, practical utilization, and effective control require a good understanding of the vibrating system A recommended way to achieve... various types, and is termed a mixed system In view of the analogy, then, a unified approach can be adopted in analysis, design, and control of these different types of systems and mixed systemsNgày đăng: 05/05/2018, 09:37
Xem thêm:
Từ khóa liên quan
Mục lục
Vibration: Fundamentals and Practice
Appendix A: Dynamic Models and Analogies
A.7.5 Causality and Physical Realizability
Tài liệu cùng người dùng
Tài liệu liên quan